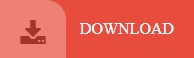
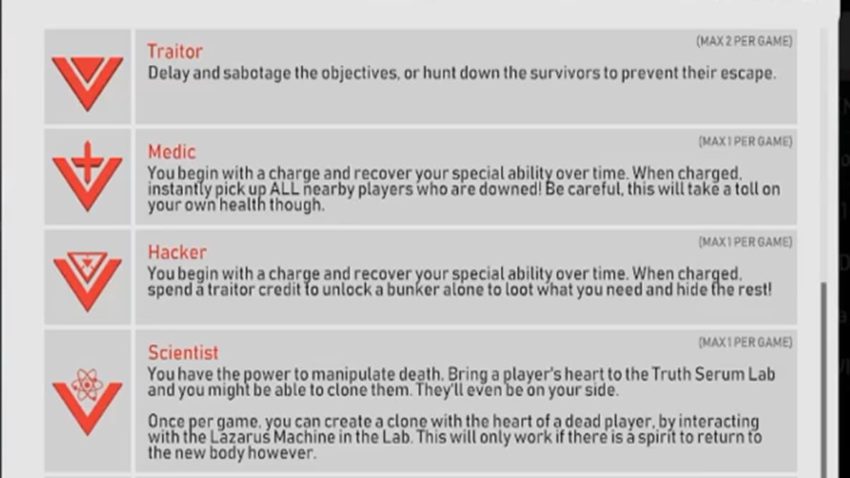
onnx(for later on-device optimization with TensorRT). tflite format(full integer and dynamic range quantization support available).
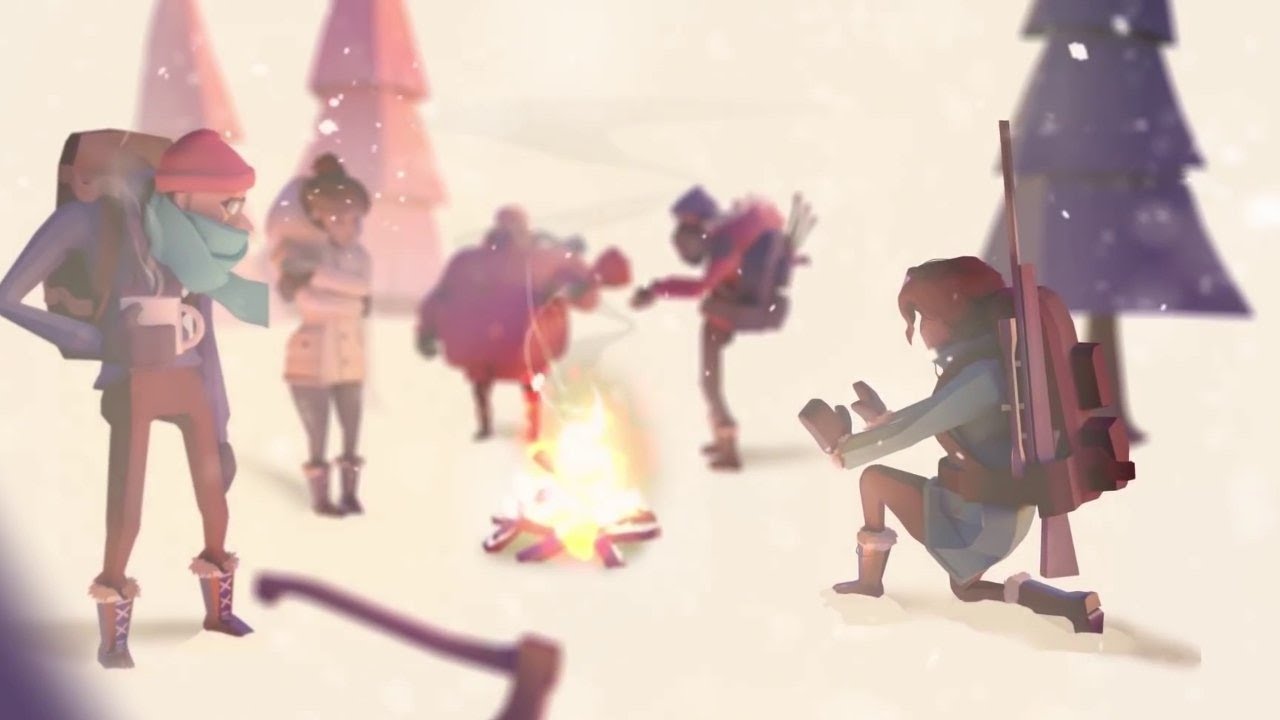
Currently supports trained model conversion to.aXeleRate will download the suitable converter automatically. Automatic conversion of the best model for the training session.Different feature extractors to be used with the above network types: Full Yolo, Tiny Yolo, MobileNet, SqueezeNet, NASNetMobile, ResNet50, and DenseNet121.Supports multiple computer vision models: object detection(YOLOv3), image classification, semantic segmentation(SegNet-basic).You put the properly formatted data in, start the training script and (hopefully) come back to see a converted model that is ready for deployment on your device! 🔧 Key Features It has easy configuration process through config file or config dictionary(for Google Colab) and automatic conversion of the best model for training session into the required file format. Human parsing Semantic Segmentation MobileNet5_0 backend + Segnet-BasicĪXeleRate is meant for people who need to run computer vision applications(image classification, object detection, semantic segmentation) on the edge devices with hardware acceleration. PASCAL-VOC 2012 Object Detection Dataset MobileNet1_0 backend + YOLOv3 Standford Dog Breed Classification Dataset NASNetMobile backend + Classifier Currently supports trained model conversion to. Mac OS/Windows are not supported) and on Google Colab.
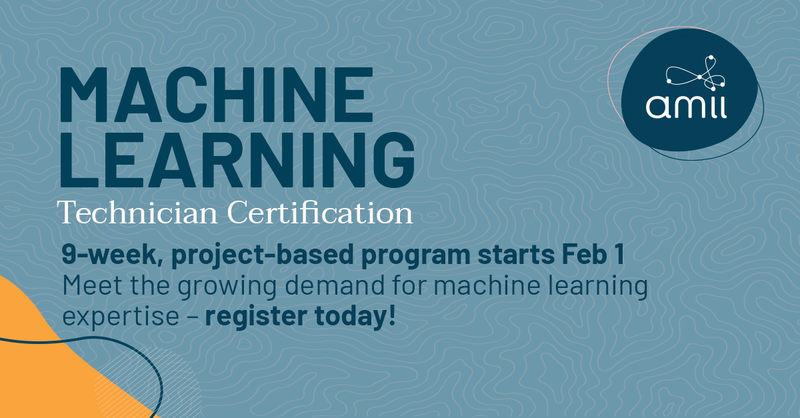
It is optimized for both the workflow on local machine(Ubuntu 18.04/20.04 - other Linux distributions might work, but not tested. AXeleRate streamlines training and converting computer vision models to be run on various platforms with hardware acceleration.
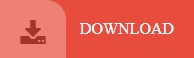